Recent trends of healthcare information and communication technologies in pediatrics: a systematic review
Article information
Abstract
As information communication technology (ICT) has advanced, the healthcare industry has embraced it to reduce medical costs, improve health outcomes, and increase patient satisfaction. Healthcare ICT revolutionizes pediatric healthcare. This study aimed to categorize and synthesize findings from the literature regarding the application of ICT in pediatric patients. This systematic review is based on a comprehensive search of Embase, MEDLINE, and Google Scholar. Study selection and coding were performed independently by 2 researchers, followed by narrative categorization. To reflect current trends in ICT for pediatrics, we adopted the Hype cycle technology classification developed by the advisory and information technology firm, Gartner, and the classification of digital health interventions by the World Health Organization. This study included a total of 135 studies. The analysis revealed 7 main types of ICT for pediatrics: (1) telehealth (39 papers), (2) precision medicine (2 papers), (3) automated decision support systems (17 papers), (4) electronic health records (7 papers), (5) patient portals (7 papers), (6) artificial intelligence (AI) (39 papers), and (7) mobile and wearable technologies (20 papers). In particular, we consistently found references to ICT for pediatrics as well as changing and improving healthcare for children. Further studies are required to determine how we can improve ICT productivity for pediatrics, particularly through AI. This study’s results will help healthcare delivery organizations and technology companies consider the future direction of pediatric healthcare.
Key message
· The innovation of healthcare information communication technology (ICT) was accelerated with the adoption of electronic health records (EHRs).
· Telemedicine currently has no technical barriers.
· EHRs and personal health records are being connected, and mobile/wearable technologies are being integrated into them.
· Conventional rule-based clinical decision support systems have already been implemented and used in EHRs and PHRs. Artificial intelligence/machine learning improves precision and accuracy.
Graphical abstract.
Introduction
The digital revolution has penetrated healthcare [1,2]. Information communication technology (ICT) for digital health has the potential to improve patient care quality, access, efficiency, and safety [3,4]. The growing demand for precision medicine and the growth of connected care between communities and hospitals necessitate a greater use of healthcare ICT for patients [5,6]. With advances in digitizing hospitals and primary care clinics through the adoption of nationwide electronic health records (EHRs), expectations that pediatric ICT will improve the quality of healthcare for children have also increased, and many studies have aimed to improve pediatric healthcare quality through ICT [7]. Previous systematic review studies attempted to synthesize information from previously published research, and ICT for pediatrics was categorized into telemedicine, EHR-related systems, decision support systems, web-based packages for patients/family caregivers, and assistive information technology to provide guidance on effective strategies for the implementation of ICT for pediatrics [8,9]. However, this categorization is old-fashioned because it does not reflect recent advancements in ICT for pediatrics, such as healthcare artificial intelligence (AI), mobile devices, and big data analysis technology. The specific objectives of this study are as follows: (1) update recent advancements in ICT for pediatrics; (2) provide precise categorization of ICT for pediatrics in significant detail; and (3) suggest a future outlook for ICT for pediatrics.
Methods
1. Design
We adopted a mixed-method systematic review that incorporated evidence from quantitative and qualitative analyses. The Cochrane Collaboration adopted it for systematic reviews to determine the effectiveness of different interventions because it is an appropriate methodology for evaluating complex phenomena in the adoption of innovations [10,11]. The method was applied based on the PRISMA (Preferred Reporting Items for Systematic Reviews and Meta-Analyses) criteria as follows: (1) eligibility criteria, (2) information source and search strategy, (3) study selection, (4) data collection and results synthesis, and (5) critical evaluation [12].
2. Eligibility criteria
We included studies that conducted a technical or clinical evaluation of ICT for pediatrics or analyzed factors influencing ICT implementation in pediatrics. Studies were excluded if they focused only on pediatric ICT design or development, related educational technologies, or clinical knowledge of ICT for pediatric patients because we aimed to focus on the practical implications of ICT for pediatric patients in the real world.
3. Information sources and search strategy
In this study, we employed comprehensive databases and web search engines, including MEDLINE, Embase, and Google Scholar. We adopted MeSH terminology. Furthermore, non-MeSH terminologies found in a previous systematic review research regarding healthcare ICT, such as “medical records systems,” “automated information processing,” “computer applications,” “computer-mediated communication,” “electronic communication,” “mobile,” “wearable,” “pediatric psychiatry,” “pediatric assessment,” and “pediatric patients,” were also used [13]. Articles published between January 1990 and January 2020 were considered.
The following terms were used:
· Information technology (information technology; medical informatics; computers; medical records systems; medical informatics; hospital information systems; internet; local area networks; telemedicine; educational technology; information systems; automated information processing; computer applications; computer-mediated communication; electronic communication; machine learning; artificial intelligence; mobile; wearable; internet of things); and
· Pediatrics (pediatrics; pediatric dentistry; pediatric nursing; pediatric psychiatry; pediatric assessment; pediatric patients)
4. Study selection
Studies were selected by 2 researchers (SYJ and KHL) according to the inclusion and exclusion criteria. First, references were found based on the titles and abstracts. When there was any doubt, the article was subjected to a full-text read. Second, the study selection was performed based on the full text of the article. All disagreements were resolved through consensus with 2 external researchers (KPH and WSC). Seven reasons for exclusion were identified prior to the review process. Cohen kappa coefficient was calculated to measure the inter-rater reliability. The overall study selection process is illustrated in Fig. 1.
5. Data collection and synthesis
Two independent researchers, SYJ and KHL, extracted data from the selected studies. In this research, we intended to assess the practical feasibility of ICT for pediatric patients in the real world because there has been excessive hype in digital healthcare. Thus, we adopted 2 practical frameworks to explore the recent trend of ICT for pediatric patients from the perspective of realworld implications: the Hype cycle by Gartner and Classification of Digital Health Interventions (DHIs) v.1.0 by the World Health Organization (WHO). We referenced 2 review articles entitled “Hype Cycle for Consumer Engagement with Healthcare and Wellness” by Gartner and DHIs v. 1l0 by the WHO. The WHO proposed the classification of DHIs to standardize the framework for categorizing the different ways in which mobile and digital technologies are being adopted. DHIs are generally targeted at public health audiences to establish a common and accessible digital health language for health program planners. Any pediatric ICT can be linked to unfulfilled needs in the real world (Fig. 2).
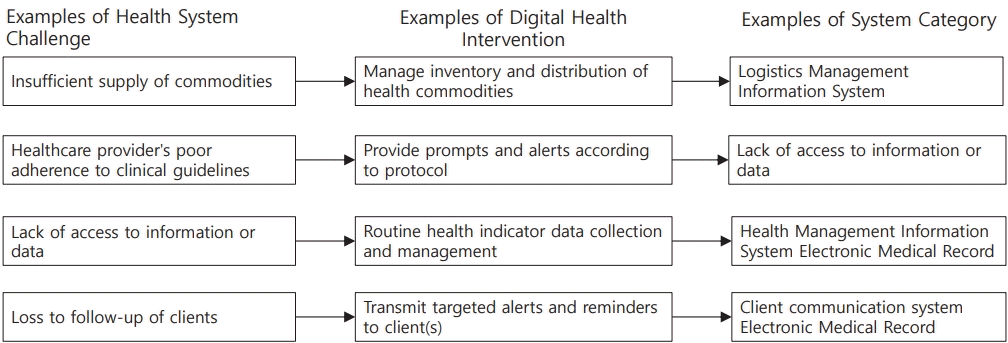
Challenges in connections across health systems, digital health interventions, and system categories presented by the World Health Organization.
We specifically adopted DHI system categories, which highlight functionalities that fit within various digital health solutions, to determine how a research topic may be fit in system categories from a practical perspective. For instance, a machinelearning algorithm that predicts the bone age of subjects can be integrated into laboratory and diagnostic information systems. Supplementary Table 1 presents the system categories of DHIs.
Hype cycles are a well-known framework for evaluating the current status of each ICT in healthcare. Fig. 3 shows a typical healthcare ICT Hype cycle [14].
We classified all included studies according to the mixed criteria of the 2 typologies.
Results
1. Characteristics of the selected papers
The primary search retrieved 1,710 papers (Fig. 1). After the inclusion and exclusion criteria were applied, 135 studies were included for further evaluation.
Table 1 presents the reasons for exclusion and the numbers excluded by category (total excluded studies: 1,432). If an article was not related to pediatrics or ICT, it was categorized as “Not related to pediatrics.”
According to Gartner classification, the included studies were categorized into virtual health assistance, precision health, AI healthcare advisors, automated decision aids, critical condition surveillance system, algorithmic medicine, Internet of Things (IOT), assistive robots, genomic medicine, patient portals and health records, population health management solutions/EHRs/health information exchange, and telemedicine solutions (Supplementary Table 2).
By combining the 2 classifications by Gartner and WHO and performing a thorough review of the selected articles, we made a mixed classification of ICT for pediatric patients (Table 2). We found that the Gartner framework did not fit in the current research fields of ICT for pediatrics because the framework views healthcare ICT from the perspective of innovation. However, we were able to explore where each research belonged in the Hype cycle from a practical perspective. System categories of DHIs from the WHO presented a detailed classification of ICT for pediatrics in terms of the characteristics of the implemented technologies.
2. Evaluation of study quality
Two independent researchers, SYJ and KHL, evaluated the quality of the included studies according to the methodology for appraising evidence in systematic reviews [15].
The evaluation process was conducted for each section of an article, and the quality was scored as good, fair, poor, or very poor. The results are presented in Table 2. Supplementary Table 3 presents the method by which the grades “good,” “fair,” “poor,” and “very poor” were assessed.
We also classified the studies that were included in this analysis by region to identify which nations were actively conducting research in ICT for pediatrics (Fig. 4). Most of the papers were published in the United States (US), followed by Europe, Asia, and South Korea.
Discussion
In this research, we followed 2 classifications that reflected recent technological advancements in healthcare ICT industries and the perspectives on how each system can be implemented for DHI. The Gartner framework was used to determine the introduction, maturity, and social application of technology from the innovation perspective. The classification of DHIs aimed to categorize the different ways in which digital and mobile technologies are being used to support health system needs. According to the mixed classification from the 2 frameworks, we found that the majority of the studies were of telemedicine (n=39 [29%]) and AI/machine learning (ML) (n=39 [29%]). Research on mobile and wearable solutions comprised 15% (n=20). Automated decision support systems comprised 12.6% (n=17). Studies of ICT for pediatrics on EHR, patient portal, and precision medicine were 5.2% (n=7), 5.2% (n=7), and 1.5% (n=2), respectively. From the perspective of the Hype cycle, telemedicine has penetrated the stage of enlightenment, in which the technology is being practically implemented. The EHRs and patient portals were at a trough of disillusionment. AI, mobile/wearable technologies, and automated decision support system technologies are still at the innovation stage.
1. EHRs and patient portals
There have been 7 studies on patient portals (5% of the total included studies). Considering the overall population’s adoption rate of patient portals, this percentage is low [16]; this implies that patient portals dedicated to pediatric patients are not common. Although the percentage is low, this finding reflects current developments in ICT for pediatrics in the provision of EHRs and patient portal solutions for patient-centered health and value-based care.
The US government launched the EHR adoption project named “Meaningful Use” (MU) nationwide in 2009. The aims of the program are to enhance data capture and exchange, advance clinical processes, and improve health outcomes by gradually introducing certified EHR technology [17]. In the final stage of MU, they aim to adopt value-based healthcare, which reduces healthcare costs and improves health outcomes utilizing EHRs throughout the healthcare process. EHRs include not only data generated in hospitals but also data generated from patients, called patient-generated health data (PGHD) [18,19].
The South Korean government is also promoting nationwide EHR quality certification programs and government-led personal health records (PHRs) that integrate public health data from the National Health Insurance System, Health Insurance Review Agency, and the Korean Center for Disease Control. In South Korea, the EHR adoption rates were 96.3% in hospitals and 95.7% in clinics, indicating that the EHR system has already reached the trough of disillusionment [20]. The EHRs and PHRs are the backbones of ICT for pediatrics. The mobiles, IOT, telemedicine, and AI technology investigated in this study aim to leverage EHRs, including PGHD, to increase diagnostic accuracy and reduce medical costs. For example, a previous study revealed that parents are more interested than their children in using pediatric patient portals, and common uses included checking medications, allergies, laboratory results, and messaging with physicians [21]. Another study proved that patient portal use can increase the perception of health state and self-efficacy as well as reduce emergency department visits and hospital admissions [22]. This finding indicates that patient portals improve the quality of care for caregivers and pediatric patients.
2. Precision medicine
Since the introduction of next-generation sequencing, precision medicine solutions have also been introduced, such as Synapse, SAP Medical Research Insights, and cBioPortal. The solutions are intended to serve as platforms to connect genomic data, EHRs, and PGHD. However, there is still room for improvement, and there are very few active solutions currently in use. For this reason, we found few articles regarding precision medicine related to ICT in pediatric patients.
In terms of integrated healthcare between ICT for pediatrics and genomic medicine, they are still in the phase of an innovation trigger. EHRs and PHRs should provide standardized phenotypic information to the precision medicine platform in compliance with standard interface design and standard terminology. For example, the All of Us program in the US adopted the standard framework of the Observational Medical Outcomes Partnership common data model for collecting and integrating EHRs into nationwide precision medicine cohorts. This is a mandatory prerequisite for integrating genomic data, EHRs, and PGHDs from PHRs because it is useless if the integrated data cannot be transferred to other countries or organizations with medical informatics standards. Thus, ICT for pediatrics should embrace precision medicine technologies based on these standards. In particular, ICT for pediatrics regarding precision medicine can be utilized to conquer rare diseases that are prevalent in children.
3. AI and ML
Several studies have been conducted to increase medical productivity using ML. ML techniques have been employed in several of these studies to predict health outcomes at the patient level with high accuracy. AI in healthcare is actively applied to radiology, pathology, disease prediction, and drug discovery. However, evidence proving the effectiveness of AI in healthcare in real-world settings remains limited. For healthcare AI to succeed, a full-cycle support system is required: big data extraction, transformation, and loading (ETL) for training and validation of AI algorithms, clinical trials evaluating the effectiveness of developed algorithms with external validation in other hospitals and organizations, certification of AI software as a medical device (SaMD), and reimbursement planning for the implementation of developed algorithms. For example, VUNO bone age, the first certified AI-SaMD, was developed under the full process from ETL to certification with one of the major hospitals in South Korea [23]. During the entire process, clinicians, AI developers, and businesspeople cooperated at every step. Healthcare professionals can actively identify unfulfilled clinical needs and solutions. AI/ML is at the stage of an innovation trigger because it is still difficult for researchers to prove the real-world efficacy of the developed algorithms. One of the reasons for this is that medical care along with medicine is conservative and path-dependent because doctors normally do not want to take risks for patient safety. However, as in the example of bone age software, the AI-assisted interpretation of bone age is more accurate and less time-consuming than human-only interpretation. This type of augmented diagnosis will prevail in clinical practice, and pediatric clinicians should embrace the AI/ ML-based decision support process [23].
4. Telemedicine
Telemedicine or telehealthcare is already at the “slope of enlightenment” stage. Particularly for children who are living at home or in nursing facilities, the potential power of telemedicine or telemonitoring is beyond expectations. For telemedicine, other technologies such as IOT, wearables, and AI-based analytics can be applied and integrated. Together with PHRs and EHRs, telemedicine can be a stepping stone for care continuum and value-based care. Since the beginning of the coronavirus disease 2019 (COVID-19) pandemic, telemedicine solutions have been growing rapidly. In the US, virtual visits through telemedicine increased from 102.4 daily to 801.6 daily (683% increase) in response to COVID-19 between March 2 and April 14, 2020 [24].
Unlike the US that has already embraced telemedicine, South Korea is one of the countries that have made illegal telemedicine. The Korean government has failed to accept long-standing requests from the healthcare industry, which would ease telemedicine regulations. However, COVID-19 has changed this situation. ICT for pediatrics should also embrace telemedicine as a paradigm shift in conventional healthcare through actual visits. Telemedicine along with EHRs, PHRs, mobile and wearable solutions, and IOT will revolutionize current volume-based healthcare in hospitals.
5. Mobile and wearable solutions
Mobile and wearable devices have been introduced to monitor physical activity in the treatment of obesity and assist in the treatment of autism. Recently, PGHD, such as lifelog data from mobile and wearable gadgets, have been integrated into EHRs because almost 50% of the determinants of health in everyday life come from PGHD [25,26]. ICT for pediatrics displays no exception to this trend. Particularly in novel virus outbreaks such as COVID-19, mobile and wearable devices together with telemedicine will become more important for monitoring infected patients without contact. Big tech companies such as Apple, Samsung, and Google have already introduced PGHD-collecting platforms. Apple Health, Samsung S-health, and Google Health are working as data exchanges and collecting hubs between mobile and wearable solution vendors and healthcare service delivery organizations. In particular, since 2019, Apple has embraced EHRs through the Apple Health app integrating hospital medical records via a health information standard named Fast Healthcare Interoperability Resources (FHIR) standard. The Office of the National Coordinator, one of the US President’s supporting groups, announced that it would be mandatory for all hospitals and healthcare companies to exchange medical information through the FHIR standard, which means that information from wearable and mobile solutions will be exchanged through EHRs and PHRs. The South Korean government launched a nationwide public PHR application in 2020. The government planned to raise the adoption rate of PHRs among healthcare consumers by opening public medical information through the app. Patients and caregivers have not been interested in PHR services to date because the information was not exchanged between hospitals and healthcare companies. However, the environment is changing rapidly, and the government is promoting patient-centered services, including mobile and wearable devices. Thus, researchers who want to improve healthcare through ICT for pediatrics should embrace mobile and wearable technology in the near future.
6. Automated decision support systems, including clinical decision support systems
Clinical decision support systems (CDSS) are already prevalent, together with the dissemination of EHRs. In particular, CDSS related to medication safety is common. However, owing to low accuracy and alert fatigue, conventional rule-based CDSS should be upgraded to meet users’ needs. ML algorithms have been adopted in CDSS to predict the risks of health outcomes at a personal level. However, the majority of CDSS are rule-based and conventional, which is why current CDSS has much room for improvement and automated CDSS is still at the stage of innovation trigger. Automated CDSS should work from end to end, with almost zero intervention from humans. Accurate and precise prediction algorithms make it possible to train themselves with real-time data and feed up-to-date results to EHRs.
The early detection of systemic inflammatory response syndrome and a decision support system for asthma are typical examples of conventional CDSS [27,28]. Automated CDSS that automatically correct errors and improve performance will prevail owing to the development of AI/ML.
7. Outlook of ICT for pediatrics
The innovation of healthcare ICT was accelerated with the adoption of EHRs [29]. With the first generation of EHRs aimed at changing the paper-based environment to a paperless environment. The second generation of EHRs utilized big data collected in the system to increase health outcomes, improve work efficiency, and decrease costs. We are still struggling to use big data in hospitals because the data were not structured based on a transferable standard format [30]. However, along with the advancement of ICT in healthcare, big data in EHRs are transforming to a standardized format. Common data models for cooperative research and FHIR for data exchange in real-world healthcare services are representative examples of such efforts [31,32]. The revolution from EHRs has been a prerequisite for innovation. We are experiencing a paradigm shift from hospital-centered to patient-centered healthcare. The former focused on the reactive management of patients whose signs and symptoms are already in the clinical stages, which means that their disease is progressing before their hospital visits. The latter is completely different. Patient-centered platforms such as PHRs with mobile, wearable devices and IOT aim at preventive management that integrates PGHD at home and professional management in hospitals. All health-related data are gathered in real time through PHRs, which are integrated into EHRs in hospitals. For example, pediatric asthma patients do not need to modify their medication doses based on patient-reported outcome measures and intermittent hospital visits. Their vital signs are monitored in everyday life using mobile and wearable technologies. The monitored data were transferred to a common healthcare platform, and ML algorithms continuously monitored the status of the data. If any aggravating condition is found, the algorithms notify the solution: modify the dosage of current medication or a virtual visit to their primary pediatrician. If the condition is checked by a doctor through telemedicine and the doctor decides to examine the patient face to face, the patient visits the hospital. Through this process, patients do not waste time or transportation costs. Doctors can see only high-or moderate-risk patients without wasting time or hospital resources. When we evaluate the current status of ICT for pediatrics from the perspective of a future outlook, we are able to understand why the Hype cycle and the mixed typology estimate the current stage of each technology.
Telemedicine currently has no technical barriers. Only laws and regulations can be barriers. However, COVID-19 is rapidly changing its negative perspectives on telemedicine. Thus, ICT researchers for pediatrics need to actively participate in the development of telemedicine solutions. AI/ML is still an innovation trigger. However, its real-world use is rapidly increasing. Clinicians should adapt themselves to a new framework that integrates AI/ML during the decision-making process. EHRs and PHRs are being connected, and mobile/wearable technologies are being integrated into them. They are at the stage of enlightenment; however, manufacturers continue to face challenges in finding services that attract consumer attention. Thus, researchers should focus on unmet needs that can be solved by ICT for pediatrics, which attracts patient attention.
Conventional rule-based CDSS have already been implemented and used in EHRs and PHRs. AI/ML will improve precision and accuracy by reducing false alarms and alert fatigue.
Conclusions
Although recent advancements in healthcare ICT have been utilized in pediatric healthcare, most are technologies for telemedicine, AI/ML, EHRs/PHRs, and mobile solutions. EHRs/PHRs have been the center of the digitalization of hospital environments, which is a prerequisite for precision medicine. AI/ML has provided healthcare professionals with accurate decision-making support systems. Mobile solutions have transformed conventional hospital-centric healthcare into patient-centric healthcare. However, there is still considerable room for improvement in precision medicine platforms, automated patient decision aid systems, and genomic medicine. In particular, precision medicine platforms equipped with AI/ML, EHRs/PHRs, and mobile solutions should be improved in the future to overcome rare diseases in pediatric patients.
Supplementary materials
Supplementary Tables 1-3 can be found via https://doi.org/10.3345/cep.2020.01333.
The system categories of Digital Health Interventions
Classification of recent pediatric ICT according to Gartner Hype cycle
Evaluation methods for previous studies
Notes
Conflicts of interest
No potential conflict of interest relevant to this article was reported.
Funding
This study received no specific grant from any funding agency in the public, commercial, or not-for-profit sectors.
Acknowledgements
Kyeongpyo Hong (KPH) and Wonseok Chung (WSC) contributed to the research and review of the papers. All the authors are grateful for this contribution.