Electroencephalography source localization
Article information
Abstract
Electroencephalography (EEG) has been and is still widely used in brain function research. EEG has advantages over other neuroimaging modalities. First, it not only directly images the electrical activity of neurons; it has a higher temporal resolution. Furthermore, current advanced technologies enable accurate mathematical calculations and sophisticated localization from EEG data. Several important factors should be considered for EEG analysis using these advanced technologies. First, raw EEG data contain physiological or nonphysiological artifacts. Therefore, preprocessing methods and algorithms to detect and remove these artifacts have been proposed and developed. In the analysis of preprocessed EEG, forward and inverse problems require solving and several proposed models have been applied. To solve the forward problem, the source information and matrix parameters from which the EEG originates are essential. Therefore, an accurate head model is required. In contrast, the possible combinations of the current sources computed inversely from EEG measured at a limited number of electrodes are infinite, referring to the inverse problem. The inverse problem can be solved by setting limits based on assumptions made of the anatomy and physiology on the generation and propagation of the current sources. Thus, methods such as dipole source models and distributed source models have been proposed. Source localization requires the consideration of many factors such as the preprocessing of raw EEG data, artifact removal, accurate head models and forward problems, and inverse computation problems. This review summarizes the methods and considerations applied to the above EEG source localization process. It also introduces the applications of EEG source localization for epilepsy and other diseases as well as brain function studies and discusses future directions.
Key message
· Electroencephalography (EEG) directly images the electrical activity of neurons at a higher temporal resolution than other neuroimaging techniques.
· EEG is still widely used in brain function research due to its advantages.
· Forward and inverse problems of EEG analyses require solutions.
· Methods such as the dipole and distributed source models have been introduced.
· Applications of EEG are expanding with the integration of other technologies and large-scale data.
Introduction
Electroencephalography (EEG) has traditionally been used to study brain function. However, brain function has also been studied using single photon emission computed tomography (SPECT) and positron emission tomography (PET). More recently, functional magnetic resonance imaging (fMRI) and magnetic encephalography (MEG) were introduced. PET, SPECT, and fMRI image secondary hemodynamic and metabolic changes in neuronal electrical activity, whereas EEG and MEG directly image neuronal electromagnetic activity [1]. EEG and MEG have the additional advantage of superior temporal resolution compared to PET, SPECT, and fMRI [1,2]. However, EEG and MEG have limited spatial resolution. Thus, many source analysis techniques have been introduced to improve its spatial resolution (Fig. 1) [1,3].
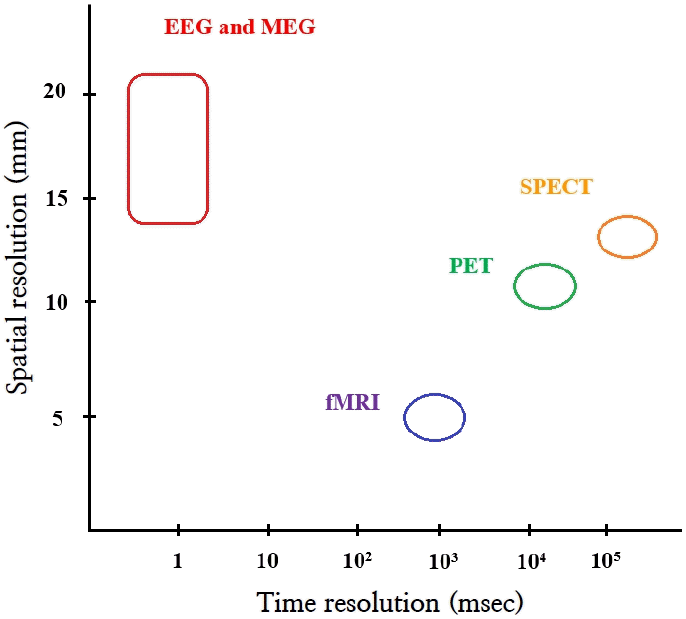
Temporal and spatial resolutions of neuroimaging modalities. EEG, electroencephalography; fMRI, functional magnetic resonance imaging; MEG, magnetic encephalography; PET, positron emission tomography; SPECT, single photon emission tomography.
EEG is still widely used in brain function research due to being much easier to implement than MEG and not requiring specialized equipment. Recent source localization methods have enabled accurate mathematical calculations and sophisticated localization from EEG data. Source localization refers to inferring the distribution of current sources from measured EEG data. However, there are many considerations in proper use of EEG for brain function studies along with recent source analysis techniques. These include the preprocessing of raw EEG data, artifact removal, accurate head models and forward problems, and inverse computation problems. This review introduces the basic concepts, technical methods, and application of source analysis using EEG.
EEG recordings
EEG, which measures the electrical activity of the brain, is divided into invasive and noninvasive EEG according to the attachment site of the measuring electrodes [4]. In the clinical and research fields, noninvasive EEG is commonly used in which electrodes are attached to the scalp by the international 10–20 system to evenly reflect activity across the brain (Fig. 2) [5,6]. The 10–20 system is the international standard used to localize the scalp electrodes. This is an important component in analyses of the localization and distribution in the source analysis of EEG. Regarding the number of electrodes used, 8–21 channels are applied in clinical practice, whereas up to to 256 channels are sometimes applied for research purposes [4,7,8].
EEG is induced by a potential difference of about 100 mV between the cell membranes of the axons caused by the excitation of pyramidal cells in the cerebral cortex [1]. These pyramidal cells are oriented perpendicular to the surface of the cerebral cortex. The summation of the postsynaptic action potentials by the action potential of each neuron is measured at each electrode through the matrix of the meninges, cerebrospinal fluid, skull, and scalp. Since the potentials measured at the electrodes are very low, the use of an amplifier to record them is required. EEG is an electrical wave that features period, frequency, amplitude, and phase components. EEG is divided into delta (0.5–3 Hz), theta, (4–7 Hz), alpha (8–13 Hz), beta (14–30 Hz), and gamma (≥30 Hz) waves according to frequency, and the recorded EEG can be considered the sum of waves with various frequencies and amplitudes [9,10].
Raw EEG data and preprocessing
EEG is visually inspected in clinical practice, through which abnormalities such as epileptiform discharges are detected and current sources in the brain are inferred. However, to accurately analyze the current sources, the potentials measured on the scalp and their coordinates should be calculated mathematically [11]. Raw EEG data are analyzed using a computer. The raw EEG file contains the potentials of each channel recorded over time. Raw EEG data can be viewed in the form of a 2-dimensional array of channel × time. These raw EEG data can also be converted into signals in the frequency domain through Fourier transformation, the decomposition of signals defined in the time domain into sine and cosine functions at various frequencies. Each sine and cosine function has its own frequency and amplitude (Fig. 3).
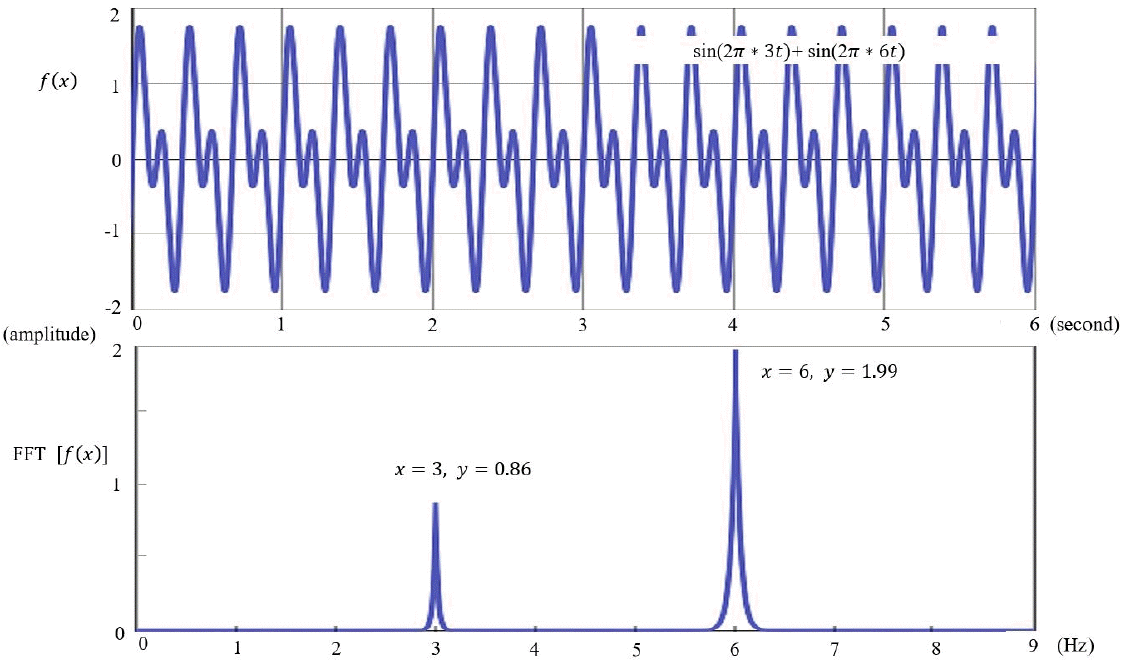
Fast Fourier transform (FFT) time and frequency domain. FFT is the decomposition of signals defined in the time domain into sine and cosine functions with various frequencies.
The raw EEG data require preprocessing prior to analysis. Raw EEG data are often contaminated by electrical activities not originating in the brain. These artifacts include physiologic (e.g., electromyography, electrocardiography, eye movements, respiration, and body movements) and nonphysiologic (e.g., electrode artifacts, power lines, and other medical devices). These artifacts should be preprocessed prior to analysis, which involves visual inspection by experienced electrophysiologists [12]. In addition, the automatic detection and removal of artifacts provided by analysis software can be implemented [13]. These preprocessing methods apply techniques such as filtering methods, wavelet transforms, and independent component analysis (ICA) [4,14-16].
Head models
The potentials and distributions in EEG measured on the scalp can be accurately determined when the initial source information and matrix parameters from which the EEG originates are known [11]. This is related to a forward problem in EEG analysis, for which an accurate head model is required to solve. Reconstruction of the head model requires variables including head shape and size and the electrical conductivity of its components (scalp, skull, meninges, and cerebrospinal fluid). Thus, an individualized magnetic resonance image (MRI) is recommended as a head model [12]. If not available, a known MRI template can be used; in such cases, the Montreal Neurological Institute (MNI template) is widely used. The MNI template uses MRI data from 152 young adults (18–43.5 years old) from the MNI by the International Consortium for Brain Mapping. However, the use of a standard template rather than an individualized MRI results in less accurate analysis results. Moreover, there are particular limitations to its use in children, for whom age-specific standard templates are required (Fig. 4) [17].

International Consortium for Brain Mapping 152 Nonlinear atlases (2009). CSF, cerebrospinal fluid; GM, gray matter; PDw, proton density-weighted; T1w, T1-weighted; T2w, T2-weighted; WM, white matter. Adapted from: http://nist.mni.mcgill.ca/icbm-152-nonlinear-atlases-2009/. Copyrightⓒ1993–2004 by Louis Collins, McConnell Brain Imaging Centre, Montreal Neurological Institute, McGill University.
Inverse problem
In the process of identifying the current sources in the brain from the potentials and distribution measured by EEG electrodes on the scalp, the number of combinations of the current sources generating the potentials and distribution measured by a certain number of electrodes may be infinite [1,11]. This is the inverse problem that can be solved by setting limitations using assumptions based on the anatomy and physiology of the generation and propagation of the current sources [1,11,18,19]. To solve this problem, many proposals have been introduced, and the proposed models and technical methods have been improved and validated [11,18,20]. Thus, the source analysis model of the dipole source localization and distributed source localization, which are currently widely used, are summarized here.
Dipole source localization
Dipole source localization was a model proposed early to solve the inverse problem in EEG analysis [1,11,18,21,22]. The cell bodies and apical dendrites of the neuron form current dipoles with opposite charges. A constraint assumed in this model is that a limited number of current dipoles consisting of anodes and cathodes at both ends in the brain produce potential fields measured by the scalp electrodes. This model is a simple and useful method that schematizes electrophysiological mechanisms of a measured EEG [23,24]. Dipole source localization is performed by calculating the location, direction, and moment parameters of the assumed number of current dipoles. The direction of the current dipole may be tangential or radial to the brain surface. Therefore, even dipoles at the same location differ in the potential field formed on the scalp by direction [11]. Given these constraints, the current source localization is mathematically solved by nonlinear optimization [21,25]. If the location of the current source is known a priori, the direction and moment of the current source can be calculated by linear optimization [21]. There are 2 approaches to dipole source localization [1,11,26]. Single time-slice localization is the method of applying the dipole source model to each time point and is mainly used for the source localization of event-related potential or epileptiform discharge [27,28]. Another approach is multiple time-slice localization, which fixes the locations or locations/directions of a limited number of current dipoles and calculates changes in the potential of current dipoles over a certain period [25,29].
Determining the number of current sources in dipole source localization is an important issue. Dipole source localization is useful if the number of current sources is known or a limited number of current dipoles can be assumed (e.g., in patients with epilepsy) [30,31]. The number of current sources can be determined by the multiple source search algorithm provided by the analysis software, which finds the appropriate number of current sources through nonlinear or linear/nonlinear optimization [18,25,32]. However, the use of dipole source localization is limited because the number of current sources is not always known or cannot be assumed [1,11]. Moreover, if the limited number of current sources is under- or overestimated, the source analysis will be biased or inaccurate [18]. However, dipole source localization is still widely used in certain situations where the number of current sources is known or can be assumed a priori [1,18].
Distributed source localization
Distributed source localization reconstructs the 3-dimensional structure of the brain into numerous lattice points (usually more than 5,000) and presents a model in which current dipoles located in each lattice are distributed in their respective strengths [11,18]. Therefore, it is unnecessary to determine or assume the number of current sources. However, the number of lattice points in the distributed source model is much higher than the number of electrodes measured on the scalp; thus, this inverse problem requires solving [1,18]. In the head model, the lattice locations are limited to gray matter and the hippocampus using individual or template MRI, and the restrictions by this anatomical information reduce the number of variables [11,18]. Moreover, in the distributed source model, a minimum norm (MN) approach is proposed that assumes the appropriateness of a distribution minimizing the total energy of each current source [33]. Here the MN solution tends to be close to the scalp electrodes and overlooks deep brain current sources [1,11]. Therefore, a depth-weighted MN approach has been proposed to compensate for this disadvantage. However, the depth-weighted MN solution features lower resolution [34-37].
Distributed source models are now widely used and increasingly sophisticated. LORETA software uses the Laplacian-weighted MN approach, which assumes that neighboring neuronal activities are correlated; thus, the current density distribution is smoothed. However, LORETA software has the disadvantage of producing blurred and over-smoothed images [38]. LORETA has been updated to sLORETA (and more recently eLORETA) to compensate for these shortcomings [39,40]. VARETA and LAURA have also been introduced by other research groups [41,42]. Moreover, a spatiotemporal source method (Beamforming) based on the assumption that neuronal activities are temporally as well as spatially correlated was introduced, and ICA was applied to localize each independent component and recombine them [43].
Applications of EEG source localization
EEG source localization has been widely used in brain research, and many studies are currently underway in various fields. More recent tools, such as fMRI, have been introduced and actively used in neuroimaging research, but EEG has its own advantages as mentioned above. In addition, the application of high-density EEG and many electrodes is readily available clinically and in research settings, and source localization methods have been further developed [12]. Therefore, EEG source localization is increasingly being used to explore brain regions associated with brain function and neurological disorders [2,18,44-46].
EEG source localization has been most commonly applied in patients with epilepsy, particularly to localize the epileptic focus in focal epilepsy [47,48]. The application of EEG source localization to presurgical evaluations in epilepsy can prove its accuracy and usefulness by comparison with intracranial EEG and surgical outcomes [49-52]. The dipole source model was initially applied to localize the epileptic focus in epilepsy surgery, and its accuracy was demonstrated by comparison to intracranial EEG and surgical outcomes [26,27]. The dipole source model has been well described, especially in studies of temporal lobe epilepsy and benign childhood epilepsy with centrotemporal spikes in pediatrics [53,54]. However, in multifocal epilepsy with multiple electrical sources, the dipole source model has limited application [55-57]. Subsequently, distributed source localization has also been applied in patients with epilepsy and was proven accurate in many studies (Fig. 5). Studies of large-scale epilepsy patients reported higher sensitivity and specificity compared to PET and SPECT [17,58]. High-density EEG and individual MRI as head models ensured this accuracy, but the application of low-density EEGs and template MRIs showed limited accuracy. Several commercial EEG analysis software packages are currently being used to localize the epilepsy focus in epilepsy surgery with intracranial EEG recording and guide the location of intracranial EEG electrodes [18].
EEG source localization is also applied in neurological and psychiatric diseases other than epilepsy [12]. Such localization has been most often applied in research and clinical studies of attention deficit disorder with hyperactivity (ADHD). ADHD is associated with abnormal frontal lobe function of inhibitory control and attention, for which EEG source localization has been applied to many studies. Several EEG source localization studies in ADHD patients have reported abnormalities in the frontal lobe and associated brain networks [59,60]. Moreover, EEG source localization plays an important role in the analysis of quantitative EEG, which was introduced and applied in clinical practice [61]. EEG source localization has also been applied in many neurological and psychiatric disorders, such as anxiety disorders, obsessive-compulsive disorder, and sleep disorders [18]. It is useful for identifying relevant brain regions in terms of neuroanatomy and physiology in brain function research. The application field is very diverse and is expanding (Table 1) [53,58,62-69].
Future directions
EEG source localization is increasingly expanding in application and continues to evolveevolve (Table 2) [70-77]. Multimodal methods, such as the integration of the high temporal resolution of EEG and high spatial resolution of fMRI, can be a promising approach. Higher spatiotemporal resolution is being demanded in neuroimaging research, and this multimodal method may be a good alternative [78]. In addition, the combination of EEG-MEG and EEG– functional near-infrared spectroscopy may provide further information in brain function research [4,79]. Along with real-time EEG monitoring, EEG source localization can be applied to the brain-computer interface, which is implemented by analyzing signals obtained from the brain and transmitting them to a computer [70,80,81]. Although many challenges require addressing, EEG source localization is expected to play an important role in brain signal analyses. Numerous neuroimaging studies are currently underway and data on EEG localization being published, but the data from these studies requires unification and integration into big data in the future, which requires the standardization of research protocols and measurement methods [71,80,82]. These tasks may be very challenging, but they can enable more advanced knowledge and technologies. A machine learning algorithm can also be applied to EEG source localization [83-85]. Such EEG source localization studies have recently been reported and are showing promising results. The introduction of these recent technologies is providing new insight into EEG research and brain function.
Conclusions
This review described the steps applied in the process of EEG source localization and their basic concepts and considerations. EEG analysis and application techniques are being increasingly developed, so EEG analysis research is expected to be more widely implemented in the future. Although the technical details of EEG analysis may be unfamiliar to clinicians, EEG source localization can provide new insight into brain function in clinical practice; therefore, increased understanding is required. Moreover, the applications of EEG source localization are expanding with its integration with other technologies and largescale data, and EEG analysis will continue to be a promising field of functional neuroimaging research.
Notes
Conflicts of interest
No potential conflict of interest relevant to this article was reported.
Funding
This work was supported by a National Research Foundation of Korea (NRF) grant funded by the Korean government (MSIT; Ministry of Science and ICT) (no. 2020R1G1A1099968).